Project Hack 7
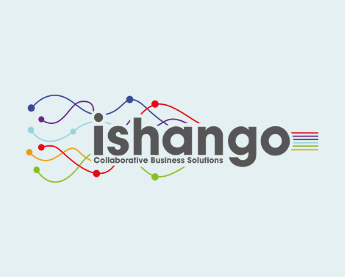
After an amazing learning experience at Project Hack 7, I felt it was the perfect time to write a blog about the weekend, what was planned and what came out of it. Firstly, the hack is organsied by Projecting Success and was completely virtual, which is an interesting experience, but it works. The Protecting Success team did a great job of organising the weekend and everything ran smooth from start to finish. The majority of the event was hosted on Discord which meant we could move around rooms freely, with masterclasses being held on Zoom for those wanting to attend them.
If you’ve never been to a hack before then it’s basically an environment where people and companies bring real world challenges, this could be anything from automatically transcribing meeting actions, analysing safety observation sentiment or even tracking close contact on construction sites. Then eager data enthusiasts select the challenge which most interests them and gather into small groups to go some way to solving their chosen challenge.
For this hack I brought my own challenge, which was to analyse data gathered from the A14 FlowForma material requisition system and try to identify the best time to purchase materials for the lowest price. If you want to read more about the requisition system, you can check out one of my previous blogs here. Now the first thing you learn at a hack is to have realistic expectations. I was using data which had over 10,000-line items, and I was lucky enough to be joined on my team by some proper data scientists. Armed with that data and data science capabilities, I envisaged the output to be a mission room which was a cross between minority report and the trading floor of the London Stock Exchange. Teams of procurement professionals plugged into the system to purchase materials at rock bottom prices.
Except, that’s not what happened, and I’ll explain why now. Firstly, although there was a good amount of data to work with, what I hadn’t factored in was the quality of the data. Only by analysing it did we realise that instead of utilising the dropdowns in the system, a lot of users had instead opted for the easier free text option. This reduced the number of data points significantly, from over 10,000 to around 6000. We then identified the key columns which would enable us to do a proper analysis of materials, their prices and any variance over time. We went through the data again and the number of data points was reduced even more to around 1200. The quality of the data had reduced the useful data points to next to nothing, which meant we couldn’t do an in-depth analysis of price variance by material over time.
We had to completely pivot from our original challenge objective and started looking at external sources to supplement our hugely reduced data set. We also used this as an opportunity to go over data quality and look at some measures which would address data quality issues.
Our challenge was now looking at external sources to pull into a Power BI report to supplement the data from the requisition system. We decided to bring in the construction tender price index, GDP and inflation figures to get an idea of causes of material price fluctuation. Another addition we made to the Power BI report was the ability to flag material prices which appeared to have been keyed in error. For example, if a material was consistently around £20 per unit and then a record said £2, you could infer that the person inputting the data had simply missed off a 0. This flag was a filter which meant that the report could be much cleaner and reduce these erroneous data points being included.
The next thing the team did was look at standard deviation for all the materials we had enough data for and indicate a high and low price for each of these materials. This was then integrated with the date the material was required so that anyone reading the report will be able to see the best price to pay for materials, as well as when it is required which means they now have the ability to decide when to pay for said material knowing exactly how many days they have until it is needed.
To sum the challenge up then, I would say be realistic with what you can achieve in just two days (that’s without factoring in the masterclasses which aren’t to be missed), work together as a team, allocate tasks, be organised and more importantly, have a load of fun solving these real-world challenges! The biggest thing which I got out of the weekend though was the importance of data quality, although you may have lots of data points, if they don’t have the key information then you may as well have nothing. Bad data is worse than no data.
Hopefully this challenge will continue to Project Hack 8, the team will regroup with the addition of some new members and we will be looking at integrating even more external sources to really build out this material pricing system and go some way to solving a real challenge from within the construction industry.